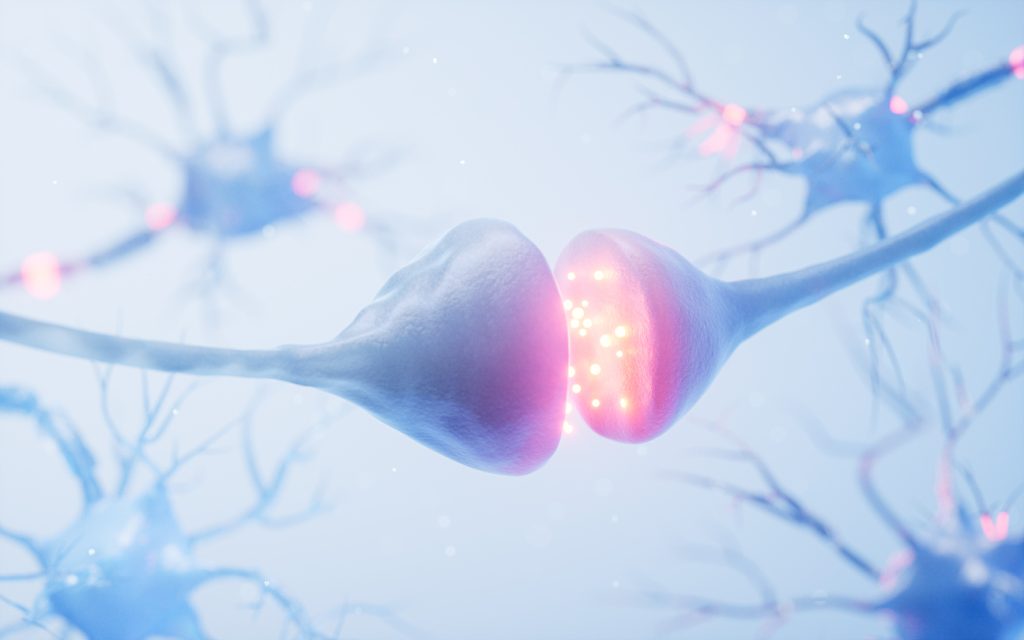
January 8, 2025 — A powerful, new framework called “Annotatability” has been developed by researchers at the Hebrew University of Jerusalem to address a major challenge in biological research — identifying mismatches in cell annotations and characterizing biological data structures.
The new study led by Jonathan Karin, Reshef Mintz, Dr. Barak Raveh and Dr. Mor Nitzan from Hebrew University, published in Nature Computational Science, introduces this method to interpret single-cell and spatial omics data by monitoring deep neural networks training dynamics. ‘Omics’ refers to the study of specific factors within a cell, tissue, or organism.
Single-cell and spatial omics data have transformed our ability to explore cellular diversity and cellular behaviors in health and disease. However, the interpretation of these high-dimensional datasets is challenging, primarily due to the difficulty of assigning discrete and accurate annotations, such as cell types or states, to heterogeneous cell populations. Genomic datasets often contain vast amounts of annotated samples, but many are annotated either incorrectly or ambiguously, making it difficult to extract meaningful insights from the data.
Annotatability identifies areas where cell annotations are ambiguous or erroneous. The approach also highlights intermediate cell states and the complex, continuous nature of cellular development.
This study demonstrates the applicability of Annotatability across a range of single-cell RNA sequencing and spatial omics datasets. Notable findings include the identification of erroneous annotations, delineation of developmental and disease-related cell states, and better characterization of cellular heterogeneity. The results highlight the potential of this framework for unraveling complex cellular behaviors and advancing our understanding of both health and disease at the single-cell level.
Borrowing from recent advances in the fields of natural language processing and computer vision, the researchers used artificial neural networks (ANNs) in a non-conventional way. Instead of merely using the ANNs to make predictions, the group inspected the difficulty with which they learned to label different biological samples.
The team then leveraged this information to identify mismatches in cell annotations, improve data interpretation, and uncover key cellular pathways linked to development and disease. Annotatability provides a more accurate method for analyzing genomic data on single cells, offering significant potential for advancing biological research and, in the longer term, improving disease diagnosis and treatment.
Researchers also introduced a signal-aware graph embedding method that enables more precise downstream analysis of biological signals as part of the study. This technique captures cellular communities associated with target signals and facilitates the exploration of cellular heterogeneity, developmental pathways, and disease trajectories.
The research paper titled “Interpreting single-cell and spatial omics data using deep neural network training dynamics” is now available in Nature Computational Science and can be accessed at here.
Researchers:
Jonathan Karin1, Reshef Mintz1, Barak Raveh1 & Mor Nitzan1,2,3
Institutions:
1) School of Computer Science and Engineering, The Hebrew University of Jerusalem
2) Racah Institute of Physics, The Hebrew University of Jerusalem
3) Faculty of Medicine, The Hebrew University of Jerusalem